External validation of a clinical prediction model for mid-term mortality after video-assisted thoracoscopic surgery lobectomy for non-small cell lung cancer
Introduction
Despite surgical resection for non-small cell lung cancer (NSCLC) being associated with the greatest chance of long-term survival, less than 20% of patients with lung cancer in the United Kingdom (UK) receive this treatment (1). This low resection rate can be attributed to either advanced disease stage at presentation [more than 50% of patients have stage IV lung cancer at the time of diagnosis (2)] or physiological unsuitability for surgical resection.
In order to appropriately risk stratify patients being considered for surgical resection, both the British Thoracic Society and the National Institute for Clinical Excellence advocate the use of a global risk score to predict short-term mortality (3,4). Whilst many models have been developed for this purpose (5), formal external validation using contemporary patient cohorts has demonstrated that none are adequate for predicting short-term outcomes in contemporary practice (6). Although a number of models designed to predict long-term survival also exist (7-9), few models are available to specifically predict mid-term outcomes, such as 1-, 2- and 3-year survival (10). Whilst no formal definitions exist, in general, short-term mortality refers to death within the first 90 days of surgery, whilst long-term survival is considered to include those patients surviving in excess of 5 years after surgery.
Thoracic surgical practice has undergone major changes in recent years, with the rapid adoption of minimally invasive procedures recognised as one of the most significant developments (11). Guidelines now recommend that all early-stage lung cancer resections should be performed via a video-assisted or robotic-assisted thoracoscopic surgery (VATS and RATS) where possible (12). A model to estimate 2-year survival specifically after VATS lobectomy for NSCLC has been developed (hereafter referred to as the Leeds model) (13). The model includes six predictors, each of which is attributed a value (either 1 or 2 points). Patients were stratified into three groups based on their aggregate scores.
Before a clinical prediction model can be widely recommended, external validation is required. The objective of this study was therefore to validate the performance of the Leeds model for predicting 2-year outcomes following VATS lobectomy for NSCLC. Pre-operative risk factors associated with 2-year mortality within our own cohort were also explored. We present the following article in accordance with the STROBE reporting checklist (available at https://vats.amegroups.com/article/view/10.21037/vats-22-9/rc).
Methods
Patients
All consecutive patients who underwent VATS lobectomy for NSCLC between January 2012 and December 2018 at Manchester University NHS Foundation Trust and Liverpool Heart & Chest Hospital were included. Patients undergoing alternative anatomical VATS resections (i.e., segmentectomy) were excluded. Prior lung resection and neoadjuvant treatment were not considered to be exclusion criteria. All cases of NSCLC were confirmed pathologically, and post-operative staging was assigned based on the post-operative histological analysis according to the 8th edition of the Tumour Node Metastasis Classification for Lung Cancer. The survival period was defined as the number of days from the date of surgery to the date of death.
Data
Our data collection methods have been described in previous publications (6). Risk factors with more than 15% of data missing were excluded. Missing categorical data were imputed based on an assumption that missingness was equal to absent, whilst missing continuous data was replaced with either the mean (for normally distributed data) or median (for non-normally distributed data) value. Such imputation strategy is likely to match how these data were collected from a clinical perspective (14). The primary outcome was 2-year mortality. Secondary outcomes were 90-day mortality and post-operative length of stay (PLOS). There were no missing outcome data. All data were cleaned and stored in the Northwest Clinical Outcomes Research Registry (NCORR) database (IRAS 260294), which has full ethical approval from the regional Research Ethics Committee of the Health Research Authority. As part of the database’s ethical approval, individual patient consent is not required as data are anonymised prior to analysis. The project was approved by the NCORR steering committee. The study was conducted in accordance with the Declaration of Helsinki (as revised in 2013).
Leeds model
The Leeds model was originally developed by entering all selected variables with a univariable P value <0.1 into a multivariable Cox proportional hazards model (13). Continuous variables were dichotomised using a receiver operating characteristic (ROC) curve analysis to identify a threshold effect for each variable. An aggregate score was subsequently created by proportionally weighting the hazard ratio (HR) of each variable retained in the final model. Age ≥75 years, percentage predicted diffusion capacity of the lung for carbon monoxide (DLCO) ≤70%, American Society of Anesthesiologists (ASA) score >2 and Performance Status (PS) score >1 were all assigned a score of one point, whilst male sex and body mass index (BMI) <18.5 kg/m2 were allocated a score of two points. Finally, patients were grouped into three classes of risk according to their aggregate score: group A (score 0), group B (score 1–3) and group C (score >3).
Statistical analysis
Continuous variables are presented as mean ± standard deviation (SD) and median [interquartile range (IQR)] for normal and non-normally distributed variables, respectively. Normality was assessed visually using histograms and statistically using the Kolmogorov-Smirnov test. Discrete variables were presented as percentages. Differences in outcomes between the Leeds model risk groups were compared using the log-rank analysis and survival curves were produced using the Kaplan-Meier method.
Multivariable Cox proportional hazards regression analysis was undertaken to identify predictors in our cohort independently associated with 2-year survival. Adjusted HRs and 95% confidence intervals (CI) were calculated. Variables were selected for inclusion based on clinical relevance and were rationalised in order to maintain an events per variable ratio of approximately 10, to minimise the risk of overfitting. No evidence of multicollinearity between covariates was found, following assessment using the Pearson correlation coefficient, the variance inflation factor and eigenvalues.
Model validation
Model validation was undertaken in accordance with the principles of validation of a Cox model outlined by Royston et al. (15). Discrimination was assessed informally by visually inspecting the Kaplan-Meier curves produced to display differences in 2-year survival between the three risk groups. A comparison of the HRs between groups was also undertaken by fitting a Cox model with a dummy variable representing each group. The absence of estimated baseline survival function in the model development manuscript meant that the ability to calculate measures of calibration was limited. However, in accordance with the methodology outlined by Royston et al., the prognostic index (PI) was calculated for each patient. An additional Cox model was fitted with the PI variable and the coefficient of the PI produced by the model represents the calibration slope of the Leeds model, with a value of 1 representing perfect calibration.
Throughout the study, the Transparent Reporting of a multivariable prediction model for Individual Prognosis or Diagnosis (TRIPOD) guidelines for reporting and conduct were adhered to (13). We also assessed the risk of bias of the Leeds model using the Prediction model Risk Of Bias ASsessment Tool (PROBAST) tool (16). All statistical analyses were performed using Statistical Package for the Social Sciences (SPSS) version 28 (SPSS, Inc., Chicago, IL, USA).
Results
In total, 862 patients underwent VATS lobectomy during the study period, of whom 42.6% (n=367) were male. The mean age was 69.1±9.0 years. Other patient characteristics are summarised in Table 1. The overall 90-day and 2-year mortality were 2.0% (n=17) and 12.9% (n=111), respectively. The median PLOS was 4 days (IQR, 3–6 days) and the median follow-up time was 30 months (IQR, 22–46 months).
Table 1
Variable | Summary (n=862) | Missing data (%) |
---|---|---|
Age, years (mean ± SD) | 69.1±9.0 | 0 |
Age >75 years | 24.7% (n=213) | |
Male sex | 42.6% (n=367) | 0 |
ASA, median (IQR) | 2.0 (2.0–3.0) | 0.5 |
ASA >2 | 31.4% (n=271) | |
PS, median (IQR) | 1.0 (0.0–1.0) | 0.6 |
PS >1 | 3.8% (n=33) | |
% Predicted DLCO (mean ± SD) | 71.5% (17.9%) | 8.7 |
% Predicted DLCO <70% | 47.1% (n=406) | |
BMI, kg/m2 (mean ± SD) | 26.8±5.4 | 6.5 |
BMI <18.5 kg/m2 | 3.2% (n=28) | |
Creatinine, median (IQR) | 74.0 (64.0–86.0) | 10.3 |
Anaemia | 18.4% (n=159) | 11.3 |
Diabetes | 15.3% (n=132) | 0.8 |
Hypercholesterolaemia | 16.5% (n=142) | 0.9 |
Hypertension | 46.6% (n=402) | 0.7 |
Smoking | 81.8% (n=705) | 1.3 |
Arrhythmia | 8.4% (n=72) | 2.2 |
COPD | 44.0% (n=379) | 3.1 |
Cerebrovascular disease | 9.0% (n=78) | 2.2 |
SII (mean ± SD) | 81.8±57.8 | 1.9 |
Right-sided resection | 60.9% (n=525) | 0 |
Resected segments (mean ± SD) | 3.7±0.9 | 0 |
T stage | 0 | |
T1 | 61.3% (n=528) | |
T2 | 33.9% (n=292) | |
T3/4 | 4.8% (n=42) | |
Any nodal disease | 11.9% (n=103) | 0 |
Thoracoscore (mean ± SD) | 1.2%±0.8% | |
RESECT-90 (mean ± SD) | 2.0%±2.0% |
SD, standard deviation; ASA, American Society of Anaesthesiologists; IQR, interquartile range; PS, Performance Status; DLCO, diffusion capacity of the lung for carbon monoxide; BMI, body mass index; COPD, chronic obstructive pulmonary disease; SII, systemic immune inflammation index, calculated as [(platelet count × neutrophil count)/lymphocyte count]/10; Thoracoscore, designed to predict in-hospital mortality; RESECT-90, designed to predict 90-day mortality.
Leeds model external validation
The aggregate Leeds model score ranged from 0 to 7 with a modal score of 1. According to the original Leeds classification (Table 2), 13.2% (n=114) of patients were in group A, 73.0% (n=629) in group B, and 13.8% (n=119) in group C. The Kaplan Meier curves are shown in Figure 1. Log rank analysis demonstrated a significant difference in 2-year mortality between the three groups (P<0.001). The HRs compared across groups were also statistically significant (group 1 vs. group 2: HR =2.412, 95% CI: 1.050–5.537, P=0.038; group 1 vs. group 3: HR =5.209, 95% CI: 2.162–12.551, P<0.001; group 2 vs. group 3: HR =2.150, 95% CI: 1.402–3.298, P<0.001). The calibration slope for the model when applied to this cohort of patients was 1.416 (95% CI: 1.334–1.896), suggesting a degree of under-estimation of risk. The risk of bias assessment deemed the model to be at high risk of bias due to concerns regarding model development and internal validation.
Table 2
Variable | % (N) | 2-year mortality, % (N) |
---|---|---|
Score | ||
0 | 13.2 (n=114) | 5.3 (n=6) |
1 | 27.1 (n=234) | 9.8 (n=23) |
2 | 24.7 (n=213) | 9.9 (n=21) |
3 | 21.1 (n=182) | 17.6 (n=32) |
4 | 10.9 (n=94) | 25.5 (n=24) |
5 | 2.3 (n=20) | 25.0 (n=5) |
6 | 0.5 (n=4) | 0 (n=0) |
7 | 0.1 (n=1) | 0 (n=0) |
Class | ||
A | 13.2 (n=114) | 5.3 (n=6) |
B | 73.0 (n=629) | 12.1 (n=76) |
C | 13.8 (n=119) | 24.4 (n=29) |
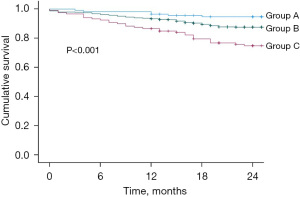
Internal risk factor analysis
The results of the Cox proportional hazards multivariable analysis identified that in our cohort advanced age, lower percentage predicted DLCO, higher systemic immune inflammation index (SII), higher tumour stage and the presence of nodal disease were all independently associated with 2-year mortality after VATS lobectomy. These results are shown in Table 3.
Table 3
Variable | Hazard ratio | 95% confidence interval | P value |
---|---|---|---|
Age | 1.042 | 1.015–1.068 | 0.002 |
Male sex | 1.363 | 0.924–2.010 | 0.118 |
ASA | 1.118 | 0.761–1.642 | 0.571 |
PS | 1.131 | 0.792–1.616 | 0.497 |
% Predicted DLCO | 0.972 | 0.959–0.985 | <0.001 |
BMI | 1.014 | 0.975–1.054 | 0.496 |
Anaemia | 0.783 | 0.477–1.285 | 0.333 |
Cardiovascular comorbidity | 1.302 | 0.844–2.008 | 0.232 |
COPD | 1.227 | 0.820–1.837 | 0.320 |
SII | 1.003 | 1.001–1.005 | 0.010 |
T1 disease | 0.566 | 0.381–0.841 | 0.005 |
Presence of nodal disease | 1.856 | 1.189–2.898 | 0.007 |
ASA, American Society of Anaesthesiologists; PS, Performance Status; DLCO, diffusion capacity of the lung for carbon monoxide; BMI, body mass index; Cardiovascular comorbidity, including hypertension, ischaemic heart disease and arrhythmia; COPD, chronic obstructive pulmonary disease; SII, systemic immune inflammation index, calculated as [(platelet count × neutrophil count)/lymphocyte count]/10.
Discussion
The previously developed Leeds model for predicting 2-year mortality after VATS lobectomy for NSCLC has demonstrated the ability to reliably stratify patients into risk groups with different mid-term survival. There is evidence that the Leeds model under-estimates the risk of mid-term mortality in our cohort and a number of additional risk factors for reduced 2-year survival were identified.
Following review in accordance with the PROBAST tool, the Leeds model was found to be associated with a high risk of bias. This was due to a number of factors including a relatively low events per variable ratio, the decision to dichotomise continuous variables [a statistically unsound approach which weakens the predictive effect of the variable (17)] and the limited reporting of measures of model performance. Whilst the high risk of bias raises concerns that a model may not perform well on external validation, that was not the case for the Leeds model in this study. Nevertheless, the issues identified may lead to inadequate model performance if the Leeds model is externally validated in alternative cohorts in the future.
Retrospective studies are limited by the quality of the data. In this study, we present a low rate of missing data and a granular dataset replete with a large number of clinically relevant variables. The size of our patient cohort was broadly similar to the patient cohort used in the development study. Whilst in the UK, larger national databases such as the National Lung Cancer Audit database are available, they collect a limited number of variables. This means that whilst use of such databases would undoubtedly increase study power, model quality would suffer due to the absence of important and clinically relevant variables not present in the dataset.
Studies attempting to stratify patients in accordance with their risk of mid-term mortality are limited. Models designed to predict and stratify patients according to the risk of short-term mortality are much more frequent, perhaps because of their alternative uses beyond risk prediction. Such tools are frequently used for risk adjustment of outcomes at surgeon-specific, unit-specific and national levels, with evidence suggesting that utilisation of risk prediction models for this purpose is an extremely effective approach (18-20).
Chamogeorgakis et al. reported a significant difference in 2-year survival on log-rank analysis between four quartiles of patients undergoing thoracic surgery between 2002 and 2006 in a single North American centre (21). Patients were separated into four groups based on their estimated risk according to the Thoracoscore model, itself originally designed to predict in-hospital mortality (22).
All risk factors included in the Leeds model were also included in the multivariable analysis undertaken as part of this work, although not in the same format. Two of the six variables retained in the Leeds model also emerged as independently associated with 2-year mortality in this study. Age is the most frequently utilised variable in models designed to predict short-term mortality after thoracic surgery (5) and has also emerged as significantly associated with adverse mid (10,23) and long-term (7,24-26) outcomes. In the Leeds model, it was dichotomised at 75, however in our analysis it remained significant when included as a continuous variable. Its retention of significance when handled as a continuous variable demonstrates its importance across the range of patient ages included in our cohort.
DLCO was a risk factor in the Leeds model and our cohort. There is evidence that spirometry values have become less important in predicting post-operative outcomes (27), whilst DLCO has emerged as increasingly important (28). Nevertheless, spirometry values feature more frequently in existing risk prediction models in comparison to DLCO. This is perhaps due to gas transfer traditionally being performed less routinely compared to spirometry, a fact which may be attributable to the higher risk of user error associated with the technique. Brunelli et al. have also previously shown that the predictive effect of DLCO was preserved when analysed solely in a population of patients undergoing anatomical VATS resections (29). The impact of DLCO on long-term survival after lung resection has also been demonstrated (30) and a number of models designed to predict long-term outcomes after lung resection include DLCO as a predictor (26,31).
A number of risk factors included in the Leeds model were not significantly associated with 2-year survival in our cohort. These included male gender, ASA, PS and being underweight. Male sex has also previously been shown to be associated with poor short (29,32) and long-term (25,26,33) outcomes. Male sex not being identified as a risk factor in our cohort may be related to the multivariable analysis including biological confounders such as cardiovascular disease, which disproportionately affect men.
ASA and PS are both subjective measures of functional status. Both measures have been included in risk prediction models designed to predict both short (5) and long-term (7,10,31,34-36) outcomes following thoracic surgery. A small number of studies have also demonstrated the ability of these measures to risk stratify in cohorts where all patients undergo thoracoscopic surgery, although this finding is not replicated in all studies (37).
Possible reasons for these risk factors not being identified as significant in our cohort include the subjective nature of the risk factors and inclusion of these risk factors as ordinal variables.
The final variable included in the Leeds model but not identified as significant in our cohort is BMI. Again, rather than utilising BMI as a continuous variable, it was included in the Leeds model as a binary variable. From a statistical perspective, this is not an unreasonable approach given both the accepted international definitions of high and low BMI, and evidence suggesting a non-linear relationship between BMI and outcomes. We explored different modelling approaches in this study, including offering the dichotomous low BMI variable to the model. However, once this did not emerge as significantly associated with 2-year mortality, we opted to instead utilise the continuous BMI variable, given our recent experience demonstrating the validity of BMI as a continuous variable in predicting 90-day mortality after all lung resections, regardless of approach (32).
We included a number of risk factors in the multivariable analysis that were not included in the Leeds model analysis. SII is a serum measure of inflammation comprised of neutrophil count, platelet count and lymphocyte count and emerged as significantly associated with 2-year mortality. This is in keeping with recent evidence assessing the impact of serum measures of inflammation on outcomes after patients undergoing resection for lung cancer, which demonstrated that measures of systemic inflammation are independently associated with short, mid and long-term outcomes (Taylor et al., unpublished).
Additionally, we also included measures of cancer stage in our multivariable analysis. It remains unclear why no staging variables were included in the Leeds model, particularly as the manuscript did include a number of univariable analyses which demonstrated superior outcomes for patients with T1 tumours, and inferior outcomes for patients with nodal disease. These results mirror our own findings as detailed in this study. Given the irrefutable relationship between advanced cancer stage and worse overall prognosis (38), we believe that measures of cancer stage should be a principal component of all tools designed to predict mid and long-term outcomes after resection for lung cancer.
Despite some of the shortcomings with the development and internal validation of the Leeds model this analysis provides some confidence that the model is suitable for use outside of the development cohort. Due to significant differences in risk factors between the studies and adequate performance of the Leeds model, we have opted not to develop a new clinical prediction model in our cohort. In our opinion future work should focus on the output from both studies to either consider refining the Leeds model or to inform the development of a clinical prediction model for mid-term outcomes that combines physiological risk factors with measures of systemic inflammation and disease stage for use in all patients being considered for surgical resection of NSCLC.
Conclusions
Whilst a number of limitations persist, external validation of the Leeds model in a broadly similar patient cohort suggests that the model could potentially be used as part of pre-operative decision making to provide additional information to patients and clinicians with regards to mid-term outcomes following minimally invasive lung resection. Future tools developed for this purpose should consider including measures of systemic inflammation and cancer stage to improve model accuracy.
Acknowledgments
Funding: None.
Footnote
Reporting Checklist: The authors have completed the STROBE reporting checklist. Available at https://vats.amegroups.com/article/view/10.21037/vats-22-9/rc
Data Sharing Statement: Available at https://vats.amegroups.com/article/view/10.21037/vats-22-9/dss
Conflicts of Interest: All authors have completed the ICMJE uniform disclosure form (available at https://vats.amegroups.com/article/view/10.21037/vats-22-9/coif). The authors have no conflicts of interest to declare.
Ethical Statement: The authors are accountable for all aspects of the work in ensuring that questions related to the accuracy or integrity of any part of the work are appropriately investigated and resolved. The study was conducted in accordance with the Declaration of Helsinki (as revised in 2013). The study was approved by the NCORR steering committee (IRAS 260294) which has full ethical approval from the regional Research Ethics Committee of the Health Research Authority. Individual consent for this analysis was waived due to the anonymisation of patient data and the retrospective nature of the project.
Open Access Statement: This is an Open Access article distributed in accordance with the Creative Commons Attribution-NonCommercial-NoDerivs 4.0 International License (CC BY-NC-ND 4.0), which permits the non-commercial replication and distribution of the article with the strict proviso that no changes or edits are made and the original work is properly cited (including links to both the formal publication through the relevant DOI and the license). See: https://creativecommons.org/licenses/by-nc-nd/4.0/.
References
- NCRAS. National Cancer Registration & Analysis Service and Cancer Research UK: “Chemotherapy, Radiotherapy and Tumour Resections in England: 2013-2014”; 2017.
- Zhang C, Wang L, Li W, et al. Surgical outcomes of stage IV non-small cell lung cancer: a single-center experience. J Thorac Dis 2019;11:5463-73. [Crossref] [PubMed]
- Lim E, Baldwin D, Beckles M, et al. Guidelines on the radical management of patients with lung cancer. Thorax 2010;65:iii1-27. [Crossref] [PubMed]
- NICE Guideline [NG122] Lung Cancer: diagnosis and management; 2019.
- Taylor M, Hashmi SF, Martin GP, et al. A systematic review of risk prediction models for perioperative mortality after thoracic surgery. Interact Cardiovasc Thorac Surg 2021;32:333-42. [Crossref] [PubMed]
- Taylor M, Szafron B, Martin GP, et al. External validation of six existing multivariable clinical prediction models for short-term mortality in patients undergoing lung resection. Eur J Cardiothorac Surg 2021;59:1030-6. [Crossref] [PubMed]
- Onaitis MW, Furnary AP, Kosinski AS, et al. Prediction of Long-Term Survival After Lung Cancer Surgery for Elderly Patients in The Society of Thoracic Surgeons General Thoracic Surgery Database. Ann Thorac Surg 2018;105:309-16. [Crossref] [PubMed]
- Kumagai S, Marumo S, Arita M, et al. Development and validation of a preoperative prognostic index independent of TNM stage in resected non-small cell lung cancer. BMC Pulm Med 2017;17:166. [Crossref] [PubMed]
- Pilotto S, Sperduti I, Novello S, et al. Risk Stratification Model for Resected Squamous-Cell Lung Cancer Patients According to Clinical and Pathological Factors. J Thorac Oncol 2015;10:1341-8. [Crossref] [PubMed]
- Balata H, Foden P, Edwards T, et al. Predicting survival following surgical resection of lung cancer using clinical and pathological variables: The development and validation of the LNC-PATH score. Lung Cancer 2018;125:29-34. [Crossref] [PubMed]
- NLCA. National Lung Cancer Audit Lung cancer clinical outcomes publication; 2019.
- Richens D. GIRFT Programme National Specialty Report for Cardiothoracic Surgery; 2018.
- Tan HX, Drake BC, Chaudhuri N, et al. A risk model to predict 2-year survival after video-assisted thoracoscopic surgery lobectomy for non-small-cell lung cancer. Eur J Cardiothorac Surg 2020;57:781-7. [Crossref] [PubMed]
- Hickey GL, Grant SW, Cosgriff R, et al. Clinical registries: governance, management, analysis and applications. Eur J Cardiothorac Surg 2013;44:605-14. [Crossref] [PubMed]
- Royston P, Altman DG. External validation of a Cox prognostic model: principles and methods. BMC Med Res Methodol 2013;13:33. [Crossref] [PubMed]
- Moons KGM, Wolff RF, Riley RD, et al. PROBAST: A Tool to Assess Risk of Bias and Applicability of Prediction Model Studies: Explanation and Elaboration. Ann Intern Med 2019;170:W1-W33. [Crossref] [PubMed]
- Altman DG, Royston P. The cost of dichotomising continuous variables. BMJ 2006;332:1080. [Crossref] [PubMed]
- Pompili C, Shargall Y, Decaluwe H, et al. Risk-adjusted performance evaluation in three academic thoracic surgery units using the Eurolung risk models. Eur J Cardiothorac Surg 2018;54:122-6. [Crossref] [PubMed]
- Brunelli A, Morgan-Hughes NJ, Refai M, et al. Risk-adjusted morbidity and mortality models to compare the performance of two units after major lung resections. J Thorac Cardiovasc Surg 2007;133:88-96. [Crossref] [PubMed]
- Brunelli A, Xiume' F, Al Refai M, et al. Risk-adjusted morbidity, mortality and failure-to-rescue models for internal provider profiling after major lung resection. Interact Cardiovasc Thorac Surg 2006;5:92-6. [Crossref] [PubMed]
- Chamogeorgakis TP, Connery CP, Bhora F, et al. Thoracoscore predicts midterm mortality in patients undergoing thoracic surgery. J Thorac Cardiovasc Surg 2007;134:883-7. [Crossref] [PubMed]
- Falcoz PE, Conti M, Brouchet L, et al. The Thoracic Surgery Scoring System (Thoracoscore): risk model for in-hospital death in 15,183 patients requiring thoracic surgery. J Thorac Cardiovasc Surg 2007;133:325-32. [Crossref] [PubMed]
- van der Pijl LL, Birim O, van Gameren M, et al. Validation of a prognostic model to predict survival after non-small-cell lung cancer surgery. Eur J Cardiothorac Surg 2010;38:615-9. [Crossref] [PubMed]
- Young KA, Efiong E, Dove JT, et al. External Validation of a Survival Nomogram for Non-Small Cell Lung Cancer Using the National Cancer Database. Ann Surg Oncol 2017;24:1459-64. [Crossref] [PubMed]
- Bria E, Milella M, Sperduti I, et al. A novel clinical prognostic score incorporating the number of resected lymph-nodes to predict recurrence and survival in non-small-cell lung cancer. Lung Cancer 2009;66:365-71. [Crossref] [PubMed]
- Guerrera F, Errico L, Evangelista A, et al. Exploring Stage I non-small-cell lung cancer: development of a prognostic model predicting 5-year survival after surgical resection†. Eur J Cardiothorac Surg 2015;47:1037-43. [Crossref] [PubMed]
- Brunelli A, Refai M, Salati M, et al. Predicted versus observed FEV1 and DLCO after major lung resection: a prospective evaluation at different postoperative periods. Ann Thorac Surg 2007;83:1134-9. [Crossref] [PubMed]
- Ferguson MK, Vigneswaran WT. Diffusing capacity predicts morbidity after lung resection in patients without obstructive lung disease. Ann Thorac Surg 2008;85:1158-64; discussion 1164-5. [Crossref] [PubMed]
- Brunelli A, Dinesh P, Woodcock-Shaw J, et al. Ninety-Day Mortality After Video-Assisted Thoracoscopic Lobectomy: Incidence and Risk Factors. Ann Thorac Surg 2017;104:1020-6. [Crossref] [PubMed]
- Berry MF, Yang CJ, Hartwig MG, et al. Impact of Pulmonary Function Measurements on Long-Term Survival After Lobectomy for Stage I Non-Small Cell Lung Cancer. Ann Thorac Surg 2015;100:271-6. [Crossref] [PubMed]
- Brunelli A, Salati M, Refai M, et al. Development of a patient-centered aggregate score to predict survival after lung resection for non-small cell lung cancer. J Thorac Cardiovasc Surg 2013;146:385-90.e1-2.
- Taylor M, Martin GP, Abah U, et al. Development and internal validation of a clinical prediction model for 90-day mortality after lung resection: the RESECT-90 score. Interact Cardiovasc Thorac Surg 2021;33:921-7.
- Birim O, Kappetein AP, Waleboer M, et al. Long-term survival after non-small cell lung cancer surgery: development and validation of a prognostic model with a preoperative and postoperative mode. J Thorac Cardiovasc Surg 2006;132:491-8. [Crossref] [PubMed]
- Fernandez FG, Kosinski AS, Burfeind W, et al. The Society of Thoracic Surgeons Lung Cancer Resection Risk Model: Higher Quality Data and Superior Outcomes. Ann Thorac Surg 2016;102:370-7. [Crossref] [PubMed]
- Kozower BD, Sheng S, O'Brien SM, et al. STS database risk models: predictors of mortality and major morbidity for lung cancer resection. Ann Thorac Surg 2010;90:875-81; discussion 881-3. [Crossref] [PubMed]
- Xie D, Allen MS, Marks R, et al. Nomogram prediction of overall survival for patients with non-small-cell lung cancer incorporating pretreatment peripheral blood markers. Eur J Cardiothorac Surg 2018;53:1214-22. [Crossref] [PubMed]
- Zhang R, Kyriss T, Dippon J, et al. American Society of Anesthesiologists physical status facilitates risk stratification of elderly patients undergoing thoracoscopic lobectomy. Eur J Cardiothorac Surg 2018;53:973-9. [Crossref] [PubMed]
- Strand TE, Rostad H, Møller B, et al. Survival after resection for primary lung cancer: a population based study of 3211 resected patients. Thorax 2006;61:710-5. [Crossref] [PubMed]
Cite this article as: Taylor M, Hashmi SF, Martin GP, Granato F, Abah U, Smith M, Shackcloth M, Booton R, Grant SW; the Northwest Thoracic Surgery Collaborative (NWTSC). External validation of a clinical prediction model for mid-term mortality after video-assisted thoracoscopic surgery lobectomy for non-small cell lung cancer. Video-assist Thorac Surg 2022;7:24.